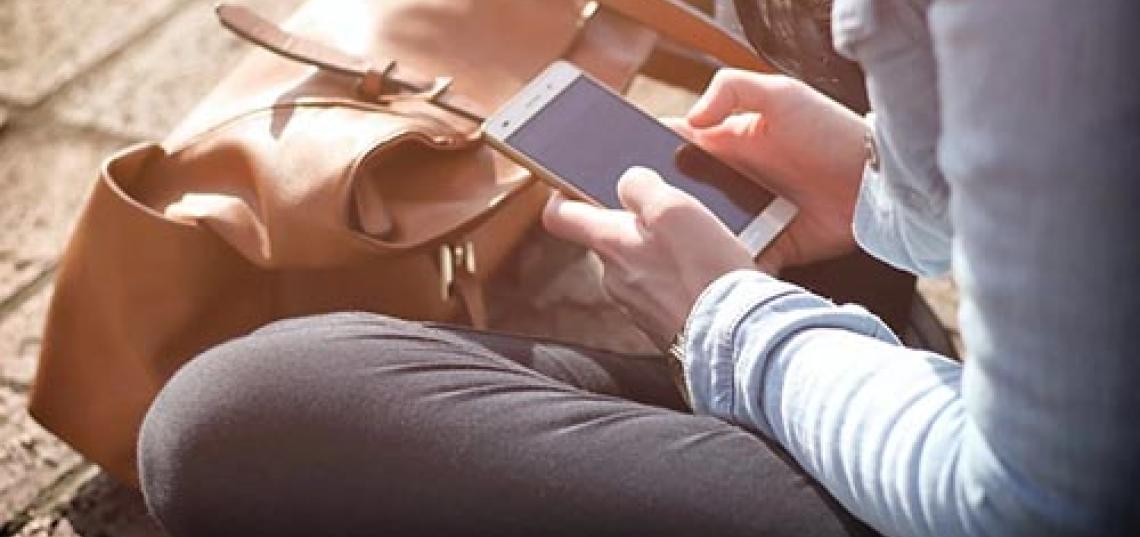
A new approach to fight bias against women in mobile phone-based mental health assessment algorithms has been proposed in a new Rutgers study that found the performance of these algorithms can vary significantly depending on gender.
The study, “Fairness in Mobile Phone–Based Mental Health Assessment Algorithms: Exploratory Study,” was published in JMIR Formative Research. Jinkyung Katie Park Ph.D.'22 is the first author and Associate Professor Vivek K. Singh served as the senior author. Other authors are Rutgers Computer Science graduate student Rahul Ellezhuthil and Rutgers School of Public Health Professor Vincent Silenzio.
“We expect results of such algorithms to influence who gets priority access to healthcare/treatment. If the performance of algorithms is worse for women, then the quality of care provided to women will also be worse,” Singh said.
Park said that their findings are critically important because they represent a crucial step in ensuring equal access to emerging healthcare services for all sections of society, and they move the literature forward on fairness in mental health assessment algorithms, particularly with gender as a protected attribute. She added, “Such results will pave the way for accurate and fair mental health support for all sections of society.”
The phone-based mental health assessment algorithms they studied, Singh said, are a type of machine learning (ML) algorithm (computer program) that utilize mobile phone data (e.g., call counts, number of messages, etc.) to automatically assign/predict a mental health score of the users. For this study, they considered an algorithm to be fair if its performance did not vary for individuals with different demographic descriptors (e.g., gender).
Biases in ML can be especially harmful if they are part of health care algorithms, Park explained, and said in this study, the authors wanted to make sure that machine learning algorithms to predict mental health do not contribute to gender disparities through biased predictions across different gender groups (male and female).
"Our findings are critically important because they represent a crucial step in ensuring equal access to emerging healthcare services for all sections of society." -- Jinkyung Katie Park P.h.D.'22
Mobile phones are now actively used by billions of individuals across the globe, and Singh said recent studies have shown that smartphones can be used to monitor an individual’s physical activity, location, and communication patterns, each of which has been connected to mental health. Hence, the automatic assessment of mental health using ML algorithms could potentially be beneficial in estimating and intervening in billions of individuals’ mental health conditions.
“Overall, we interpreted the results to imply that it is often possible to create fairer versions of algorithms,” Park said. “However, given the variety of fairness metrics that can be considered and the complexities of practical scenarios, the process of bias reduction is likely to involve a human-in-the-loop process and consideration of the trade-offs in terms of multiple metrics. Hence, rather than identifying a silver bullet solution, there might be opportunities for multiple small modifications that allow fairer versions of the algorithms. Having said that, value-sensitive design needs to be an important part of the future design of similar applications and algorithmic audits need to become an essential step in the process of medical approval of newer (algorithmic) diagnostic tools.”
Singh said their study does not differentiate between (biological) sex and (socially construed) gender, so future studies should include participants with nonbinary gender identities, consider larger data sets, protected attributes other than gender, and a newer approach to creating fair and accurate mental health assessment algorithms.
Learn more about the Library Science and Information program and the Ph.D. Program on the website.
Pictured: Jinkyung Katie Park Ph.D.'22 and Library and Information Science Associate Professor Vivek K. Singh.
Banner image credit: Pexels